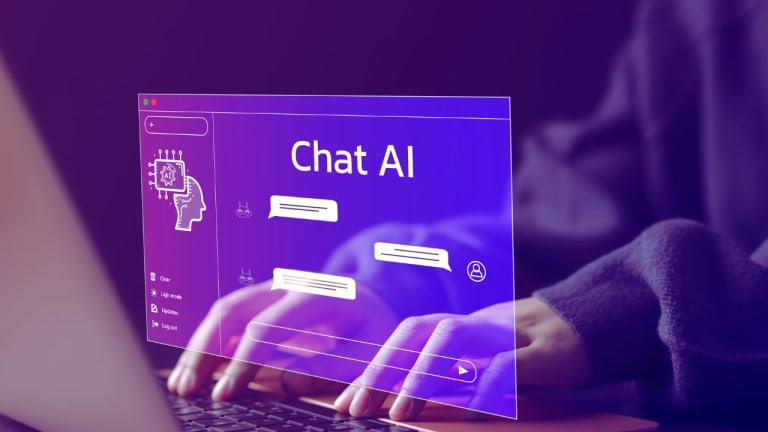
Researchers launch $50 model to take on OpenAI’s O1 – Here’s what you need to know
Technology#HRTech#HRCommunity#Artificial Intelligence
A team of AI researchers from Stanford and the University of Washington has unveiled a new reasoning model, dubbed s1, that reportedly competes with leading artificial intelligence models, including OpenAI’s o1 and DeepSeek’s R1. The most striking aspect of this breakthrough? It was trained for under $50 in cloud compute credits—a fraction of the millions typically required to develop high-performing AI models.
The research team trained s1 using a technique called distillation, a process that extracts reasoning abilities from an advanced model by training on its responses. The researchers started with an off-the-shelf base model and fine-tuned it using Google’s Gemini 2.0 Flash Thinking Experimental. This approach is similar to a recent experiment by Berkeley researchers, who built a comparable model for approximately $450.
The s1 model, along with its training data and code, has been made publicly available on GitHub, marking a significant step towards AI accessibility and open research. However, this achievement also raises concerns about the rapid commoditization of AI technology. If small teams can replicate powerful AI models at a minimal cost, it could disrupt the competitive landscape and challenge the proprietary advantages held by tech giants like OpenAI, Google, and Meta.
The affordability and efficiency of the s1 model highlight an emerging challenge in the AI industry: the erosion of exclusivity. Historically, cutting-edge AI models have required vast resources and proprietary data to achieve industry-leading performance. But as researchers refine techniques like distillation and supervised fine-tuning (SFT), the cost barrier for developing advanced AI continues to drop.
SFT, the method used in training s1, allows AI models to learn from a curated dataset of specific behaviors rather than relying on massive-scale reinforcement learning. The researchers compiled just 1,000 question-and-answer pairs, including reasoning sequences from the Gemini 2.0 Flash Thinking Experimental, to enhance s1’s capabilities. The entire training process took less than 30 minutes on 16 Nvidia H100 GPUs, with the required computing power rentable for as little as $20.
This breakthrough comes amid growing tensions over AI model replication. OpenAI has previously accused DeepSeek of improperly harvesting data from its API for distillation, raising ethical and legal questions about the boundaries of AI training. OpenAI is also embroiled in copyright disputes in India, where publishers have accused the company of using proprietary data without authorization to train its models.
As AI research becomes increasingly open-source and cost-effective, tech giants may find it more challenging to maintain control over cutting-edge innovations. While s1’s development demonstrates the potential for small teams to build competitive models at minimal cost, it also raises questions about the long-term sustainability of proprietary AI development.
While distillation methods allow for the replication of existing AI models, experts caution that they may not lead to significant breakthroughs in AI capabilities. Instead, these techniques primarily serve to democratize AI access, making powerful models available beyond corporate research labs.
As major players like Google, Microsoft, and Meta invest billions in AI infrastructure, small-scale innovations like s1 are proving that significant advancements can be achieved with minimal resources. Whether this marks the beginning of a new era of open AI development or fuels further conflicts over proprietary AI remains to be seen.